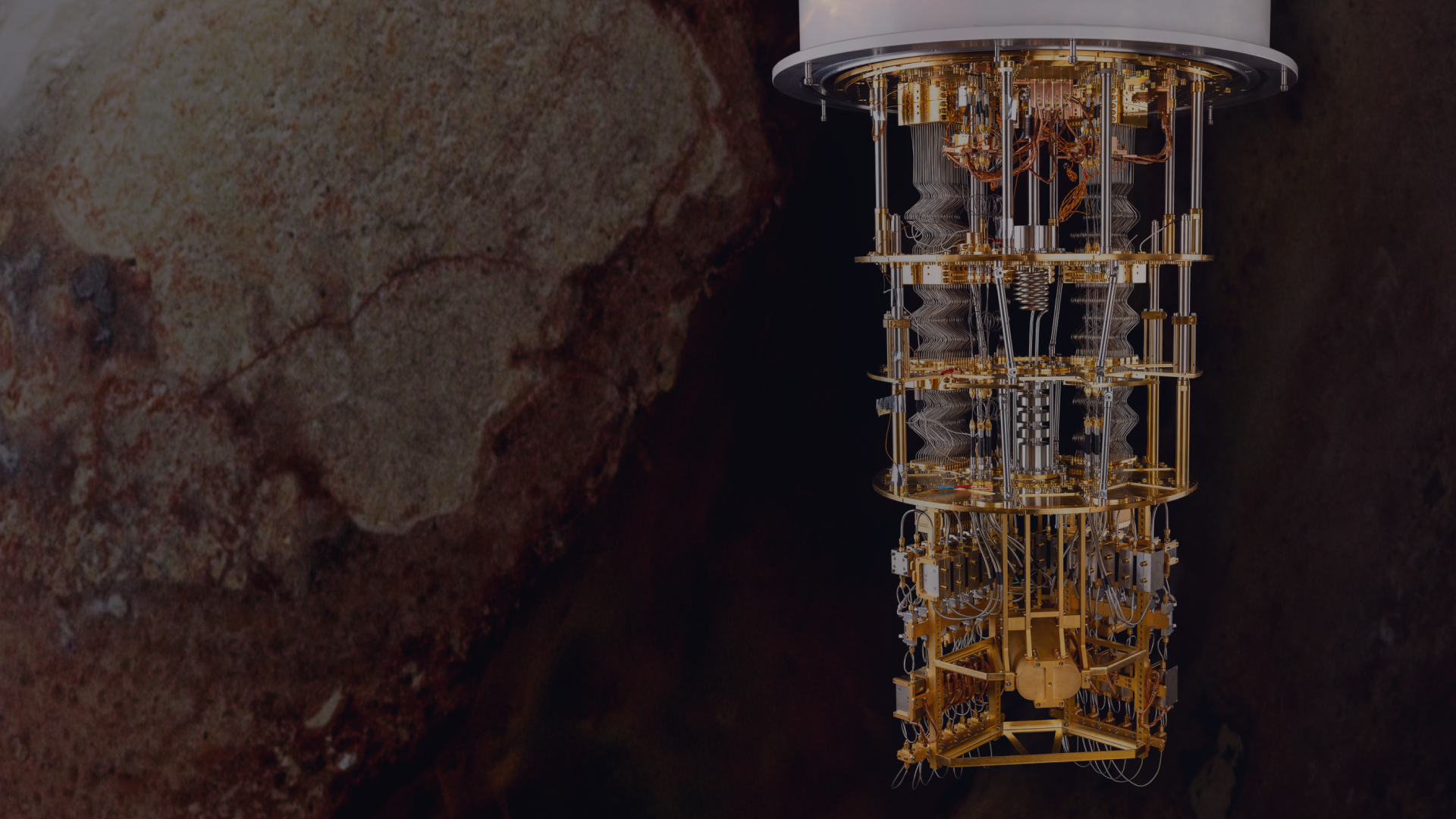
Q-CTRL Error Suppression is Now Available on Rigetti’s Ankaa-3 Quantum Computer
June 24, 2025
Exploring the biggest opportunities for quantum computing and how Capgemini is using Fire Opal to deliver advantage to businesses.
The goal of driving quantum computing forward is to deliver tangible value to enterprise. Today’s quantum computers with over 100 qubits have been shown to have problem-specific advantage. But delivering this advantage to businesses is blocked by one critical challenge: error and decoherence.
In this webinar, Dr Michael Hush, Chief Scientific Officer at Q-CTRL, is joined by Dr Sam Genway, Head of Industries from Capgemini’s Quantum Lab. We will explore the biggest opportunities for quantum computing, and how Capgemini is using Q-CTRL's error suppression product, Fire Opal, to deliver advantage to businesses.
Michael Hush
,
Chief Scientific Officer
,
Q-CTRL
Sam Genway
,
Head of Industries
,
Capgemini
Dr. Michael Hush 0:03
Hello, everybody. Welcome to Q-CTRL’s webinar on Fire Opal. So today we're going to talk to you about achieving quantum business advantage with automated error suppression. I'm Michael Hush. I'm the Chief Scientific Officer at Q-CTRL, I’ve been with the company five years and have been looking over the research program on improving the performance of quantum computers and developing the next generation of quantum sensors. And I have with me here today, Dr. Sam Genway, he's the head of industries from Capgemini's Quantum Lab. Sam, would you like to tell us a little bit more about what you do?
Dr. Sam Genway 0:39
Thanks very much, Michael. And thank you for the kind invitation to join you in the webinar. So my role within Capgemini's Quantum lab is very much focused on leading a research team, which is focused on going after some of the applications, which are of interest to our our clients and also engaging our clients on the start of their quantum journey. Really spread across sectors. So it's a fascinating role to be in - a little bit of research focus, but also getting out there and talking to people about the sort of challenges that quantum will one day solve.
Dr. Michael Hush 1:15
Excellent. So, as a few more of attendants come in, first of all, welcome. We also have with us here, Michael Warren, who is the director of our enterprise sales from Q-CTRL. He'll be jumping on later to talk more about that as we cover the Q & A.
Dr. Michael Hush 1:29
So today we will go to the webinar. We're looking to go for 45 minutes. It's going to start with a discussion with me and Sam about where we see quantum computing currently, the potential for it to have an impact on business, and what is the thing that is holding it back? What are the things that limit us from getting the most of these devices and how can we solve that? After our discussion, if you have any questions as you're going along, please put them in the Q & A, because we'll then have a short presentation around Fire Opal, our product from Q-CTRL, and improving the performance of real devices. And then after that, we'll finish with a Q & A session. Where you’re welcome to ask whatever you like about quantum computing, how to improve it, and how to use it to better your business. Please put those questions in the Q & A function. If you go down into the Zoom interface, move past that chat button and click that Q & A one, you're welcome to put questions there at any time and we'll be sure to get to them at the end. At the end, once you've finished, there will be actually an opportunity to win three $50 Amazon gift cards. All you have to do is complete the survey, which will be sent to you as an email afterwards, where you can fill in and tell us a little bit more about the experience and how we can improve these webinars going forward.
Dr. Michael Hush 2:53
So with that start, let's jump into it. Sam, what are the use cases for quantum computing at Capgemini that you've been exploring recently and you're really excited about?
Dr. Sam Genway 3:03
So, we've been in this game for a little while. Actually, most of the things that people have thought about doing with a quantum computer, we've explored a little bit, but an area we're particularly excited about is chemistry and material science. And that sort of spans applications from areas like carbon capture, battery design, right through to pharmaceutical development. And one of the reasons for that excitement in this area is that this is one of those areas where you need really, accurate simulation. You you really hit a brick wall with classical computing, and quantum computers really promised to take us past that with quantum algorithms.
Dr. Michael Hush 3:43
Yeah, that's really interesting because we once did a survey with our early user base of what algorithms they were looking at, back when we used to both be academics who actually worked together at the University of Nottingham. Back in the day, quantum chemistry was talked about as this big application. But when we did a lot of research, we found that a lot more people were concerned with quantum optimisation and the quantum Fourier transform when they're actually running on devices. It's great to see Capgemini is really making that dream of these advantages you can get in quantum chemistry a reality. So, can you dive a little bit more into the specific business cases you've been looking at and where you're seeing a potential for advantage?
Dr. Sam Genway 4:24
Yeah, absolutely. It’s sort of interesting that you talk about how people are exploring a few algorithms and how the landscape has changed a little bit in terms of what people are interested in. I think we've seen that as well. One of the things that particularly interests us is how a quantum algorithm can fit into an existing workflow and how it can add value to that. If we take an area like drug discovery, which is an area we've done some work on very recently, one of the things that's really commonplace today are data driven pipelines, the use of AI, the use of machine learning to make predictions. And one of the things that we've been looking at is how we can put feature sets, which can be calculated on a quantum computer, into a pipeline like that. So that decisions can be made based on predictions, but at the core of that, there are calculations which are quantum mechanical. So, in other words, extracting some information about a piece of chemistry potentially interacting with a piece of biology in this context.
Dr. Michael Hush 5:28
Yeah, yeah, I see. We’ve been doing work in logistics as well, and this is the challenge, right? You’ve got these incredibly big, complex problems which people have spent so much time developing a solution for. So I totally see this advantage where you really have to find that particular choke point where a quantum improvement might give them a real advantage. And rather than trying to take it all at once, we’ve been looking at optimisation of transport problems and logistics problems. Similarly, we found that if you try to tackle the full problem, it's called a capacity vehicle routing problem, it's very challenging. But in regular computers, when you try to solve this kind of CVRP thing, if you have more than 200 customers it can take days to solve it exactly. So you want to use these approximate algorithms, which are 1 to 3% less efficient than the true exact algorithm, which offers this opportunity to potentially get a better solution. When we're talking about a better solution, you might be able to route your packages more efficiently to your customers so your vehicles consume less fuel and they get them more reliably. We were looking at that problem and we were trying to pull it apart. Similarly, when we applied quantum computing, we found that it was best for this first stage where we wanted to break these many, many customers into clusters of localised people. We found that max cut, in that particular case, was a useful algorithm to first soften the problem, and then we would feed it into optimised cost. So it's like that nature of using quantum computing to help on that initial problem.
Dr. Sam Genway 7:11
I think there's actually a real parallel with some of the chemistry work we've been doing. There are certain classes of problems where correlations between the electrons in a system, a piece of chemistry, or a piece of material science play a real role. That’s where it becomes very tough for the approximate methods that we normally use on an HPC techniques, like density functional theory, to recover all of the chemistry that's interested. But at the same time, we know that for the foreseeable future quantum computers are not going to be massive. So it's really about how can we find something out about a relevant part of the problem. In some of the work that we've done recently, that's been targeted covalent drugs. These are drugs that form strong covalent bonds with biological targets, and that makes for very potent drugs. But the part of the chemistry which is relevant is that reactive part of the molecule. And that's the bit you really want to focus your attention on. Where does the quantum computer come in? Well, we know that quantum computers are good at simulation. How can we extract relevant features? We’ve been looking towards algorithms which enable Hamiltonian simulation. These are algorithms that you enable the time evolution of a quantum system, a fragment of a molecule, a bit of a material, and that gives very rich information about that system that we've actually shown can be fed into a data driven pipeline. And sure, we're at small scales today. We're in an exploratory phase. But that has the potential to be really valuable in terms of making useful predictions. And maybe that's not necessarily just replacing a classical algorithm. Maybe in the future, that's replacing an experiment with a computational technique instead, which is quite a meaningful step when we get there.
Dr. Michael Hush 9:02
So you've you've really isolated that part where you get the biggest benefit, and surprisingly, it's around simulation of those quantum dynamics themselves so it's great to see that connection forming. Let's talk about how you're running it on a real device and getting this on to a quantum computer. So you mentioned quantum computers aren’t that big, what experience have you had trying to get this component of your algorithm running on a real device? And was that of value to your analysis?
Dr. Sam Genway 9:30
It’s a really good question. I actually think the value question is a really interesting one in these times where we move from real real toy problems into an exploratory phase where, as we enter an era of quantum utility, we're really starting to focus in on “What will those problems be? Where there's that 1st advantage and when are we going to hit it?” And you could say, “Well, OK, I'll wait until there's the next generation of quantum computers and just use that.” But, for us in Capgemini we have a partnership with IBM, and we see the end to end journey is very important for a couple of reasons. I think one is, just because we have to be exploring the hardware now to really get that kind of understanding. Because it only makes sense to really be exploring this end to end. If you stop at a theoretical point about what quantum algorithms you could pick up for a particular problem, I think you learn less. But I think also kind of slightly less tangibly, it makes it all much more real in terms of the conversations we're having, where you can actually really run these algorithms. See what comes out. And actually, there's a real sort of, I'm trying to put my finger on it, but there's a real sort of warm feeling of, actually, making contact with this hardware in the early stages and doing it for real. It's no longer a theoretical exercise. So for that reason, yeah, the end to end journey is really important to us.
Dr. Michael Hush 11:05
When we've been doing applications and looking at devices, the other element we've found really useful is just getting a real understanding of where current performance is. Because we want to be getting these algorithms running on bigger devices quicker. It really helps to have an understanding of what are the challenges that real machines have right now. So you can do the research on the right area to get that algorithm running more efficiently. The simplest thing we've always considered is it's not always just the number of bits. We've been discovering for a lot of these devices it’s really about the depth, for instance, that you really have to think about as a challenge and a limitation that you have to work around in terms of, getting it running on a real machine.
Dr. Sam Genway 11:48
So I think for the question of “how do you use the quantum resource that that you have today and are likely to have in future?” It's absolutely going to be key for that nearer term. Sorry, I interrupted you.
Dr. Michael Hush 11:58
No, no, no. I was just going to ask. We obviously started, working together again because you were looking at applying Fire Opal to improve the performance of that real device. Could you tell me a little bit more about that challenge you were facing and what you are looking for Fire Opal to solve?
Dr. Sam Genway 12:17
I certainly can share a bit more about that and talk a little bit about the actual journey of how we are going to use it. We started out by doing a little bit of benchmarking and looking at inverse QFTs and things, and a member of the team had a background in quantum verification, so had done a bunch of this stuff. It was a sense of “OK, well, we're seeing these improvements in fidelity”. And that's really cool when you actually see this is something that really works and does what you want to do.
Dr. Sam Genway 12:54
Our next step was then to apply it on a project. And actually, we applied it the first time to this project in drug discovery where we were looking at extracting features from Hamiltonian simulation. You’ll be pleased to hear, there are some results from using Fire Opal in our manuscript with one of the big pharma companies. And in that, we found that using Fire Opal was the single biggest thing we could do to push getting good results in Hamiltonian simulation at small scales. And I know there are a bunch of tricks we can apply, but it was one of the things that was really nice to see. It was the single, biggest step change for an easy “take something off the shelf and apply it and see what we can get out of the hardware”. And so we included that as a demonstration from our point of view of where things are, in terms of what's possible with the hardware, when you pick a tool up like Fire Opal.
Dr. Michael Hush 13:59
So the particular application you were looking at was simulating Hamiltonians, which is always a good choice, because it's such a efficient thing to do with a quantum computer. So what was the particular challenge you saw which Fire Opal fixed? Was it was the errors that you were seeing? What was blocking the actual application?
Dr. Sam Genway 14:21
We were doing Hamiltonian simulation to create feature factors, so we weren't actually directly interested in, per se, the electronic dynamics. But actually, this gives a signature that allows us to compare to similar but different molecules. And actually, we found that we could extract features from the exact Hamiltonian simulation, which were predictive of things like reactivity and enabled us to cluster molecules with respect to their quantum properties and all such things like that. So this was really cool. But one of the challenges is that you need to evolve the electrons for long enough to see these effects appear. And, of course, evolving for longer means means that you need to have a certain circuit there, you need to have a certain number of gates in there. That's where I think the real impact is - really in being able to push that out a little bit further. I mean, essentially, there are energy scales in these problems associated with the chemical structure, which you have to be able to unpick by simulating for long enough times. And so, that kind of circuit depth element is absolutely key for us here.
Dr. Michael Hush 15:35
Yeah. Great. So, in order to get those real signals of the features that you need for it to be effective, you need that extra depth, which Fire Opal was able to to provide.
Dr. Michael Hush 15:46
So I'll jump into Fire Opal now and then we'll ask some more questions. We'll talk a bit more after I get back, because I think it's a good point to tell people a little bit more about how it works. Great, I'll just start the presentation. So, Fire Opal is a product that we’ve released at the end of last year and we've been trying it with specific customers, but it's now available for everyone to use. So what Fire Opal does is that it solves a really central problem currently in quantum computing. And that's when you try to take an algorithm, run on a real device and what you discover is an open secret, so to say, that all quantum hardware that's available today for commercial use often has so many errors on it that it tends to fail more than it succeeds.
Dr. Michael Hush 16:49
So a good example of this is shown on the screen right now. You can see what we've tried to do is execute Grover search, a classic algorithm to run on a quantum computer where you're looking to get the speed up on the search and the identification of a bit string. So here on that top plot to the right, we can see we're expecting to get a particular bits string or a particular solution with the highest probability. But then when we go and run it, the real data we get off the machine, and this is real data that's been taken off a device which has been run with bare metal errors, so no additional configuration whatsoever, we see on the bottom that you do not get the correct answer. Instead you get a a very different histogram, which has some additional structure, and you see these other peaks to the right, but they don't necessarily mean anything. It's basically all noise. This is a real challenge because these errors are occurring because quantum hardware is really susceptible to errors because it has to be engineered to such small precise, low temperature, physical physical devices. Sorry, that was not a very good explanation for someone who spends doing physics all their life. But the key thing is that quantum quantum machines desperately want to decohere and lose their coherence, and we've got to fight against this to make them work for longer times. So as a user, what that actually means for you is that when you try and execute on a quantum computer, you'll often have experiments that fail. You'll often be confused about the mismatch between your theory and the actual performance coming off the device, which can lead to confusion and a waste of resources. But to improve this, you need to really have a deep understanding of the physics of the device in order to effectively design controls and other techniques to remove these errors and fix your system.
Dr. Michael Hush 18:41
So what Fire Opal does is it provides a completely automated, error suppression solution, which can remove the majority of noise on these devices and get you to the correct solution quicker with less resources. What you can do with Fire Opal is you can use it today, and it will automatically integrate it to your workflow so that you get the right answer when you execute an algorithm. You can easily deploy it. It's deployable through Python and simply takes one line of code to actually execute. By using Fire Opal, you can save time and resources first and foremost by reducing often the number of calls that's required to get to the right solution because you're getting higher quality outputs. And also you're not wasting your time executing circuits, which may not give a correct answer. In fact, Fire Opal has inbuilt warnings and guards to tell you if you're trying to execute something on a real device, when it's well past its coherence time or it's well past the time where you're gonna get a good result.
Dr. Michael Hush 19:44
This is an example of us using Fire Opal today on that same problem I presented earlier where we're looking at Grover search. So as we can see on the left there, when I execute this on a real device, normally it doesn't give the right solution. But when I add Fire Opal, I take that same programme, pass it through our Python API and send it onto the device compiled with our controls. What happens is that it executes, and the errors are dramatically reduced. You can see here on the right, this is real data taken from a machine executed with file and we can see the correct solution now comes out as the highest probability. It’s showing you the change in the device, basically from producing noise to working. So the actual boost that you can get and be seen is that when you're using over 12 to 13 qubits, with benchmarking that we've done with the Bernstein–Vazirani algorithm, for instance, we've seen factors of over 1000 in terms of the probability of getting the correct solution compared to, what comes off with the bare metal errors.
Dr. Michael Hush 20:50
So how does Fire Opal achieve this? Well, it's a fully automated error suppression pipeline. We start with tools which would transpile and take your abstract algorithm and then most efficiently find the best qubits on the particular device to execute on the day where you’re going to get the best answer. So we actually find a mapping of where the best qubits are. We then do transpilation. So we basically figure out how to efficiently, take those abstract gates and compile it into the individual actual gates that are executed on the device, and our compiler is state of the art. They would then incorporate our controls at the circuit level. We actually use our knowledge of, robust control techniques to actually change the way that the individual gates are implemented so that when there are errors that occur on the device, our techniques are designed to take advantage of the knowledge we have with the physical system to ensure that those errors actually cancel out with themselves. In using that technique, we can ensure that when you execute it in a single execution without the need for multiple repetitions, ou can actually eliminate the errors on your device. Then, we take those gates and we tweak them and optimise them to get them to the perfect the highest, operating fidelity that we can. And finally, we have measurement mitigation techniques to ensure that any errors that are introduced at the measurement stage are fixed as well. So once that entire pipeline is automated, you don't have to worry about configuring any of it. All you have to do is hit fireopal.execute and the code will automatically run all of those control techniques and then send you the improved solution.
Dr. Michael Hush 22:36
Here is an example of what it actually looks like. So I've come here to the Fire Opal quickstart guide. So this is the documentation, you can see this on our website. First of all, it gives you instructions of how to install Fire Opal. It is as simple as signing up on our web page and then downloading a local, Fire Opal package with your Python installer. Once you have that set up, you can run and design your circuits however you wish. Here is an example where the circuit has been created in OpenQASM 2. That's the format we use, but you could very well develop it in Qiskit or PyQuil, whatever you want. You could just develop your circuits in your own environment, and then when you're ready to execute, it is as simple as just doing. Then change it to the OpenQASM 2 format and send that to the Fire Opal pipeline.
Dr. Michael Hush 23:30
Here's an example where we're going to be executing a Bernstein–Vazirani algorithm. So this is a sketch of a series of CNOT gates that occur after creating a series of superstitions at the top. When we actually want to execute that circuit, all we have to do is set up our information about our provider. In this case, we're going to be running this on IBM, but other backends will be available soon in Fire Opal. Then all you have to do is - we can obviously check that things are going to work - but when you actually want to execute it, it really is one line. So with fireopal.execute you simply send the circuits that you want to run (this is the abstract representation of your circuits), the number of shots that you want, your information about your IBM device, and which particular device on IBM you want to run it on. You don't have to say anything about compiler configuration. You don't have to put anything there whatsoever about the controls that are applied. All of that is automated. All of that is done out of the box, so you don't have to worry about it. And what is returned from this function is the actual measurement results. So you can then analyse these results and plot them.
Dr. Michael Hush 24:41
Here the Bernstein-Vazirani algorithm is looking for a particular hidden string in a function. When it works, it should return the correct string with a probability of 100%. Here we can see that when we execute Fire Opal, we do, indeed, see the correct answer with the highest probability. So we have correctly executed the circuit and got the right result. If we compare that to running it on the commercial device with bare metal, so no Fire Opal, and just using it as the default configuration out of the box. Here you can see we're just executing, in this case, using Qiskit without any additional information. You can see that the same circuit does not produce the correct bit string with the highest probability. Instead we get a different mode, and we also get some structure which is not reflective of the reality of the actual algorithm that we're trying to execute. That shows you that it really does improve the performance of a real algorithm when you run it. And also it's very easy to use. You can run it straight out of Python, and it works out of the box.
Dr. Michael Hush 25:51
So what is one of the big differences that I talked about with Sam earlier? One of the key changes that Fire Opal allows is that you can run much deeper circuits than you normally would be able to. This can be incredibly important when you're trying to get the correct answer or extract that accurate feature you need from your quantum chemistry simulation. You need to be able to execute deeper circuits. What I'm showing here on this plot is that you can execute more valuable algorithms because using that same cost of execution, you can successfully implement a circuit with a much deeper gate. You can see here we get relatively small errors, on the order of up to hundreds of gates on the actual device itself. Comparing this to running with the bare metal results, we can see over 80 gates or so (this is a a logarithmic scale) the quality of your result has dropped so dramatically that you're unlikely to see the correct answer at all. One key element of what Fire Opal does is it allows you to execute deeper circuits and gain much more valuable insight from the algorithms you're trying to run.
Dr. Michael Hush 27:18
So we've just heard from, Sam who's at Capgemini. But we've also been working with a variety of different other companies. For instance, at Parc and Quantscient. Parc says, “Our experience with Fire Opal was amazing. It achieved 4.5 times more accuracy compared to the baseline. The experience clearly indicated how much cheaper quantum computing can be when using the right tools.” We can see that by using the right tools, you can much more efficiently get to the right answer and you'll get more value for money with the money you've already invested in your device access.
Dr. Michael Hush 27:52
Just to finish up what Fire Opal really does is it allows you to execute deeper circuits, so it can execute 10 times the depth of circuits that was normally available on a device. There's a factor of 100 in terms of savings and simplicity, so you can get state of the art performance from devices, which would normally, when using bare metal errors, have a significant reduction in performance. This can allow you to use cheaper devices and get the same result or it allows you to execute with fewer runs in order to reach the same fidelity of answer, resulting in significant savings up to a factor of 100. Finally, in terms of the actual performance of the probability of boosting the correct answer from being returned from an execution, we've seen performance improvements of a factor of over 1000 using Fire Opal.
Dr. Michael Hush 28:51
I’ll stop the presentation there and get Sam to join us again. I might also just have a quick sip of water as I've been talking for 30 minutes. Everyone's welcome to jump onto the Q&A. If you go to the bottom of the screen, there's a Q&A button down there. Just click it and submit your questions. I'd also like to ask, Michael Warren to jump on. Hey, Mike. Great to have you on board. So, Mike is our Director of Enterprise Sales, and he'll be helping field some of your questions today.
Dr. Michael Hush 29:26
So we've got our first one from Bryan. “Are there limitations on how large of a device Fire Opal can be run on? If so, are these fundamental limits?” I might take that one first. At the moment the limitation is basically what devices Fire Opal has been calibrated for. I believe the 20 to 27 qubit device, from IBM is available today, or if not soon. And we're working towards having more than 100 qubit devices available on the platform as well, so there's no fundamental limit here. In terms of scaling the calibration routines that we use, scale linearly when it comes to the actual, execution of how many calibration routines we have to do in order to calibrate the full device. We can do that because, a lot of the calibration routines can be run in parallel. Just a question for you, Sam. What have been the size of devices that you're looking to actually execute on in order to see a difference? Have you done some estimates around that? I know it's a big question to ask.
Dr. Sam Genway 30:36
Yeah, it's a good question. The truth is that we're not quite in the era where we're able to push to situations where there is advantage yet. There's often a bit of a trade off in terms of qubit number versus fidelity. And actually qubit numbers are often not the issue, so we haven't always been on the larger devices. But I wondered, building off that question, whether I could have fired a question back at you in this area. I've got my own answer to it, but I think it's an interesting one. As we move from this era we are today, how do you see the role of Fire Opal? Do you see it as a NISQ era thing? What's it’s continued role? I would love to get your perspective on that.
Dr. Michael Hush 31:31
That's a good question. The long term vision is that we're looking to accelerate quantum advantage. So we're looking to bring it here quicker, faster and earlier with these NISQ era early devices, which are smaller scale. Before error correction, we're quite confident that the techniques we’ve built in there will make those devices more useful to more users earlier by allowing the execution of deeper circuits more reliably. But our long term vision for Fire Opal is to start incorporating things like a simple error correction to begin with. As these devices start to have a capability of being able to do mid-circuit measurements and feedback, for instance, dynamic circuits are available on IBM, that's looking to be more reliably released to all customers. Next year, we're looking to take advantage of that and start doing elements of feedback to improve performance, heading towards full-fledged error correction when that will provide a benefit on a real machine. We're always looking to add new features, and we're looking to add new features which will improve the performance of devices. As a new technique arrives and becomes relevant, whether it is another error suppression technique or it is error correction we will integrate it and allow it to work.
Dr. Michael Hush 33:03
I think I've got another question from Liz. Over to you, Mike. “What industries will benefit the most from quantum computing capabilities?”
Michael Warren 33:14
Well, that's a great question. What we're seeing is a cross-industry penetration of quantum computing. If you take a topic like financial optimisation or supply chain optimisation using QAOA, you're seeing that across industries. That is good for the financial area, pharmaceuticals, materials science, transportation, and government. So you can't really think of it as a vertical, but think of it as a horizontal with tentacles coming down and looking at various tractable use cases. Great question, but we are seeing it across the spectrum of verticals.
Dr. Michael Hush 33:51
That's an interesting point, that you're seeing the same algorithm or the same technique being useful in multiple industries. Sam, do you want to also add a comment on a bit more about these quantum chemistry opportunities or anything else you've been looking at?
Dr. Sam Genway 34:05
Even taking a step back from quantum chemistry, I agree completely. It's across many verticals. It's interesting that the drivers are sometimes different across different industries. Sometimes you'll see an industry where R&D cycles may be quite long, and therefore, it really makes sense to be thinking about this now. Because if you think about the life cycle of R&D, then you know by the time one cycle is finished, we may be in such a further advanced state here. In other cases, it may be if you don't get involved now, then what are the risks in not being ready for this technology as it as it evolves. So I think there are potentially slightly different kind of motivations across across different industries. But I would agree completely that this absolutely spans multiple verticals.
Dr. Michael Hush 34:56
There's this idea of being ready for when a quantum computer is of a sufficient size and quality to give an improvement. It’s a really important thing to be aware of, I believe. There’s also a good reason why you want to be trying things on devices now so that you have a understanding of when that's going to arrive.
Dr. Michael Hush 35:16
We have another question around getting started. So, the question is, “How do you get started? Say, I have a quantum algorithm that runs fine on a simulator but only gives noise on a quantum computer. I would love to test this.” So, first things first, go try out Fire Opal. All you have to do is go to https://q-ctrl.com/fire-opal. There you can get started immediately to improve an algorithm that you're trying to run on a real device. So you do need to have device credentials, but you can get that through IBM. Once you have that, you can work with Fire Opal to get an improvement. We're talking more broadly, though. So, if you have an algorithm that runs well on a simulator, but only gives noise on a quantum computer, what would you recommend, Sam? What would be your advice for, making progress?
Dr. Sam Genway 36:33
I think there's near term and longer term things here. Certainly, looking to the toolbox of tricks and as you've already said, there's the answer. But what I'd also throw into the mix is that if you've discovered an interesting use case and even with all of the tricks that can be thrown at it today, it's still not possible to get something working. Then also remember that the growth and the development of hardware is really quite rapid. It's encouraging when you see encouraging results on a simulator as well, and to come back in six months time and see what you can run then with Fire Opal.
Dr. Michael Hush 37:30
Yeah, for sure. There'll be new devices work with Fire Opal, and new, better machines are being released every year. So it’s very much worth keeping an eye on.
Dr. Michael Hush 37:39
Another question we have from Bryan, “Can you describe the types of error mitigation that are used and possibly how they are different than some of the basic techniques built into Qiskit?” This is around Fire Opal, so I'm happy to answer.
Dr. Michael Hush 37:51
We have, our own error suppression techniques, which are compiled at the circuit level. These are similar in spirit to dynamical decoupling, where dynamical decoupling allows you to self-cancel noises in your process. But what we do differently in Fire Opal is we compile our robot sequences with an understanding of what gates are being executed, and that makes a significant boost in performance. That’s compared to the basic techniques which are built under Qiskit, where Qiskit allows you to deploy very simple decoupling sequences. In terms of performance, we still see a really significant gap. We've done testing with 16 qubits for Bernstein Vazirani and we've seen that factor of 1000x improvement in error mitigation and suppression techniques in Fire Opal compared to one which has been pre-configured in Qiskit. The other comparable pieces: Qiskit has a compiler. Our compiler is fully pre-configured and outperforms Qiskit's default setting. You'll also see a significant improvement in the compilation. Finally, our error mitigation has is also 50% better in its error mitigation effectiveness compared to what is built into Qiskit. So there are head to head technical advantages in using what Fire Opal has, but outside of that, you don't have to configure it. So that's also a really significant win. Often what is available in Qiskit requires a lot of specialised knowledge to get working and that can take a lot of time and resources. Obviously, learning something requires a lot of actual executions and that can slow you down. I was just going to ask Sam, did you ever try any other of these error mitigation techniques that are available in Qiskit or other options before trying Fire Opal?
Dr. Sam Genway 39:55
Oh, we did certainly explore those as well. To be clear, of course, we certainly see the value of those techniques. As I said earlier, one of the things that we did - and I don't want to claim that we've done an absolutely exhaustive look at all of them - but one of the things that we did in our most recent work was to pick out the different options and see the impact they had. We even have a plot in the in the paper showing the counts of Pauli strings and compares all of those, and Fire Opal does the best as a single tool to get the best possible result. So this was very pleasing for us to find.
Dr. Michael Hush 40:49
OK, great. Mike, do you want to highlight any other calls that you've had with people and where they've seen a real difference with Fire Opal and what it can provide to their applications?
Michael Warren 41:01
Absolutely. What we're seeing is another trend that's out there, and it's really moving across enterprises, academia, and military. People want to see what their job runs like on an actual quantum computer. And what people are seeing is as these machines ramp up in the number of qubits and their capabilities, quantum scientists will be getting a real feel for looking at their intractable jobs and seeing what kind of results they can get at various qubit levels. That will allow them to plot in a graph the degree of difficulty in solving these problems. As the, the number of qubits increase in the machine, you'll see the usage really ramp up, especially on the, the IBM 127 qubit machine. Then, the real benefit of Fire Opal will come into play there for everyone to see.
Dr. Michael Hush 42:00
Great. We’re heading to the end of our plan here today. I just have one question for both of you. What would be an application that you're really excited about for quantum computing, which you don't think gets as much coverage as you think it should have? I'll go to you first, Sam.
Dr. Sam Genway 42:21
Given the conversation, the answer is obvious. I don't think that the interesting bits of material science and chemistry are getting as much attention as all the people talking about optimisation and quantum machine learning and all of these things. I obviously have quite a bias. I'm a many-body physicist by training and these problems really excite me and I really want to see us move across to. They require a sort of niche understanding because not everybody is experiencing them every day. But their impact will be huge. So there's, for example, the impact on on the sustainability challenges, which could be really, really big. So that's why banging on around batteries and carbon capture is something I'm going to keep doing.
Dr. Michael Hush 43:15
Excellent. Mike, do you have another one?
Michael Warren 43:17
Sam, I think that's great. And the impact on the long term will be very dramatic and everyone will benefit. In the shorter term, if you go back to QAOA looking at optimisation and looking where enterprises can really get value out of quantum computing using Fire Opal, just look around supply chain optimisation. If a company or an organisation has a multibillion dollar supply chain and you're able to optimise that by three quarters of 1% year over year, you're talking about a big number. That's something the CFOs of organisations can hang their hat on and go, “Wow, this is something we really need to look at. This is good for the business. And here's the ROI that goes with it.” I think we're quickly getting there. In the next couple of years you'll start to see a real impact in that area.
Dr. Michael Hush 44:10
I totally hear you on that. That would probably be my answer. Although we, at Q-CTRL, look most at optimisation, I am really fascinated about seeing the actual execution of quantum Hamiltonians for something useful. That’s really interesting to me because it's something that we talked about for years as researchers, that this is going to be the application. But it felt like it fell away from the industry for quite some time. So I'm really interested to see this coming back and also with IBM’s utility demonstration.
Dr. Sam Genway 44:50
It’s very timely in terms of challenges like that. Clearly, the impacts are also huge if it can happen. There are so many use cases, like battery design, where if you can improve the storage capacity by a small amount, given how ubiquitous they will be across the globe, it’s a huge impact in terms of our wider sustainability goals. There are lots of examples like this where, as we start to see the tipping point, it gets really exciting across industries and use cases.
Dr. Michael Hush 45:29
Thank you both for joining us today. So thank you, Mike. Thank you, Sam. And thank you, everyone else who, attended the webinar and asked questions. Once again, there's a survey that will come at the end of this. If you fill it out and let us know how we did and what you'd like to see more in the future, there are 3x $50 gift vouchers in the mix for it. But otherwise, if you are looking to run a real algorithm, if you're looking to explore how your algorithm performs on a real device so that you have a better understanding of when it's going to have an effect for quantum advantage, please go to https://q-ctrl.com/fire-opal. You can get started with Fire Opal today and deploy it and see improvements to algorithms that you want to run on real devices. Thank you again, everybody, and hope to see you all soon at the next webinar.
Read the latest company news, quantum research, and product updates from Q-CTRL.
Learn how Q-CTRL provides the critical software to help you do more with your hardware.
Background material and research developed by the Q-CTRL team.
Learn the most common terms and frequently asked questions in quantum computing and quantum sensing.
Keep pace with quantum technology straight to your inbox