Setting a new milestone towards an efficient and sustainable quantum-enhanced supply chain






Transport and logistics face complex computational challenges that require immense processing power to solve. McKinsey estimates that quantum computing could have an economic impact of as much as $63 billion by 2035, and the supply chain and logistics industry is positioned to be one of the early benefactors of quantum technology.
In supporting partners like Transport for NSW and the Australian Army, we have demonstrated our capability to address industry-relevant constrained optimization problems using quantum computing. Recently, we have also developed a quantum solution for a complex manufacturing supply chain problem involving minimizing costs and carbon emissions as part of the Airbus BMW Group Quantum Mobility Quest.
Quantum optimization of a real-world supply chain problem
In response to our team’s exceptional performance in Phase I, the judges replaced the simplified model with a real-world scenario to provide an opportunity to deliver actual value with a quantum solution. The challenge posed in Phase II is computationally intractable to solve exactly - obtaining the globally optimal solution would take tens of thousands of years to compute!
Imagine the complexity of an aircraft supply chain and its composite parts. Here we needed to address a supply chain optimization problem by allocating product components to manufacturing locations under specific constraints:
- Supply chain resilience: A dual-sourcing approach where each part is produced in two locations in different countries, ensuring production is not halted if the supply chain is disrupted in one location.
- Transportation feasibility: For each part, there must be a transportation route connecting the production and destination location.
- Location capabilities and capacities: Each location has different manufacturing resources capable of producing different parts. The profitability of each location must be guaranteed by location-specific minimum workshares and the individual maximum production capacity cannot be exceeded.
- Supplier workshare: Orders cannot fall below supplier-specific minimum workshare constraints. Dependence on each supplier is limited by individual maximum workshare constraints. Each supplier also has a target workshare that is considered ideal.
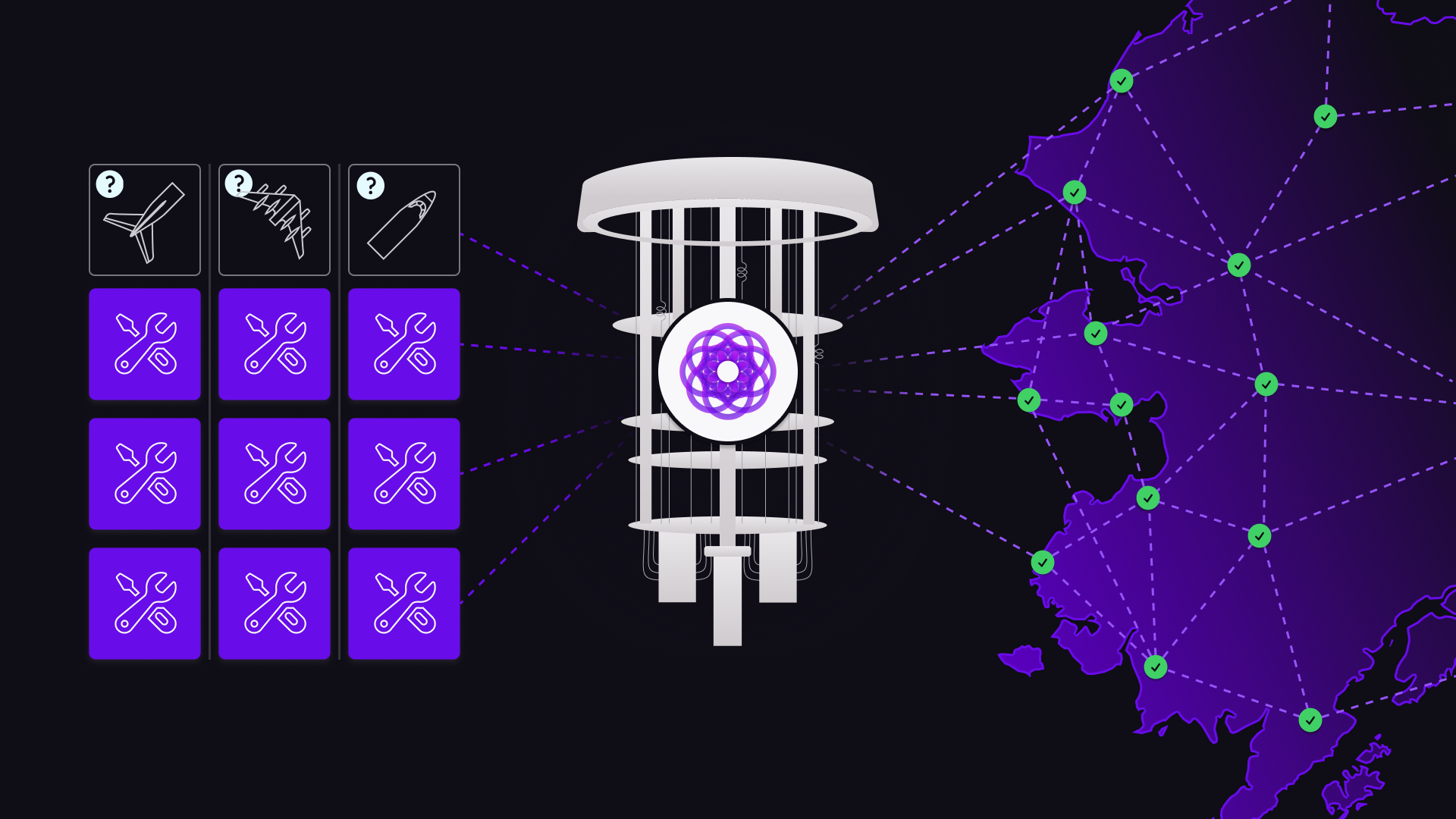
This problem is particularly challenging as allocation decisions are highly interdependent. For example, a change of location for the final assembly of the aircraft simultaneously affects the workshare of the corresponding locations and suppliers, requiring rerouting of the many subparts for the final assembly. Additionally, due to the finite capacities of the cargo containers, each transportation option has a different marginal cost - sometimes we can add another cargo unit to a transported batch or we might have to consider an additional shipment in other circumstances.
Applying a hybrid quantum computing workflow
Since the full optimization problem is of a size and complexity that makes it impractical to address directly, we designed a computation workflow based on a Very Large-Scale Neighborhood (VLSN) search procedure. This means that the full supply chain problem is decomposed into a sequence of assignment subproblems to be solved iteratively. Even these subproblems are computationally intractable: we apply quantum optimization to pursue high-quality solutions quickly. Our approach is a heavily modified version of the Quantum Approximate Optimization Algorithm (QAOA), a key feature of our Fire Opal performance-management software.
We successfully deployed our quantum optimization at up to 98-qubit scales on hardware, achieving the optimal solution in every instance! Even at this large scale, our solver provides a high probability of measuring the optimal solution, and we expect high quality results from our solver for even larger assignment subproblems.


We compare to a simple "greedy" local search: if the quantum hardware was overwhelmed by errors our solver would match this, and therefore we can see that our custom algorithm and error-suppression pipeline produce strong performance on the hardware.
We then matched the quantum solver’s resource requirements with IBM’s and IonQ’s published hardware roadmaps. Based on these roadmaps, we project that our quantum optimizer will be able to obtain high-quality solutions for our target of a 48-product real industrial problem, considering 5 candidate options per product, in 2028.

Reaching a new milestone toward useful quantum optimization
We’re thrilled that our quantum solver, adapted to this particular constrained problem, works exceptionally well - a new milestone in our progress toward useful quantum optimization!
We found that our solution is limited by the hardware size, not by the quality. We performed experiments on IBM and IonQ (via Amazon Braket) and, based on their publicly available qubit and gate-count roadmaps, we expect to be able to optimize the full-scale challenge supply chain problem in the next few years. We are excited to assess the quantum solution quality at this computationally-challenging scale, as we push towards quantum-enhanced solutions in an industry-critical domain.
We thank Airbus and BMW Group for hosting the challenge, which we believe has provided an ideal candidate problem for exploring early value from quantum optimization. The challenge has also allowed us to demonstrate the world’s largest successful quantum optimization of a constrained problem on gate model quantum computers!
With our solution, quantum optimization for relevant and useful industrial problem sizes is within sight. Contact our expert team today to discuss how we can help solve your optimization challenges.