Exploring the future of quantum-powered logistics with Airbus and BMW Group




Imagine a future where improved logistics management - powered by quantum computing - plays a key part in reducing carbon emissions across industries. We’re excited to say we’re working hard to make this a reality.
Recently, our team was named a finalist in the Quantum Powered Logistics category of the Airbus and BMW Group Quantum Computing Challenge. The challenge is part of a global initiative that aims to develop quantum computing technologies that could solve some of the biggest challenges faced by the aviation and automotive sectors.
Our project focused on real-world problems faced in energy-intensive manufacturing industries. The problem we chose to focus on in the first phase of our effort involved optimizing the allocation of parts to manufacturing locations, aiming to minimize financial and emissions costs in transportation of the parts during the assembly process. This problem stood out to us not only because of its substantial economic and environmental benefits but also because it aligns perfectly with our expertise in developing hardware-tailored quantum computing solutions for logistics optimization.
It's a true thrill to be one of just three finalists in this category from over 100 original submissions to the competition. We’ll tell you more about what was so unique about our approach and entry below!
A hybrid approach toward realizing quantum advantage in optimization
The challenge that we considered was defined by a “product breakdown structure” which is a supply-chain diagram showing how a complex product (such as an airplane or car) is built up from component parts, which themselves are built up from component parts, and so on. Given a limited range of specialist manufacturers and dependencies between how parts are assembled, the problem is to figure out the best place to manufacture each part, while abiding by the restriction that — imagining the product breakdown structure as a family tree — no two parts having a parent-child or sibling relationship can be manufactured in the same location. The definition of “best” here is the solution that has the minimum possible carbon emissions from all the transportation in assembling the product.

The first thing we did was to prove that this problem posed by Airbus and BMW is actually efficiently solvable classically. With the right strategy, you can find the best place to manufacture each part extremely quickly, even when there are lots of parts and manufacturing locations. So, we looked at a harder version involving the allocation of each part to two manufacturing sites, introducing redundancy into the supply chain.
Even though this seems like a relatively minor modification, we were able to demonstrate that this can make the problem classically intractable, and we then set out to solve it with quantum computers!
The key to our approach was centered around a problem decomposition strategy, where we noticed that finding the best manufacturing location for the final part is a matter of first finding the best manufacturing locations for each of its component parts, and then combining these smaller solutions in the right way to get the best solution for the part itself. By starting at the bottom of the product breakdown structure diagram, and building up our solutions level-by-level to the top, we solved the problem far more efficiently than trying to tackle the whole thing at once.
For this version of the problem, it can in some cases be really hard to find the best way to combine the child-part solutions into the best parent-part solution. It’s these sub-problems that we identified were best suited to the quantum processor.
Our methodology involved a customized version of the QAOA Solver available in Fire Opal and incorporated additional prototype features. The approach we used to ensure that our solutions obeyed the parent-child and sibling manufacturing location restrictions resulted in a remarkable improvement in runtime efficiency compared to standard methods: it’s subject to a patent application (patent #WO2023097358A1).
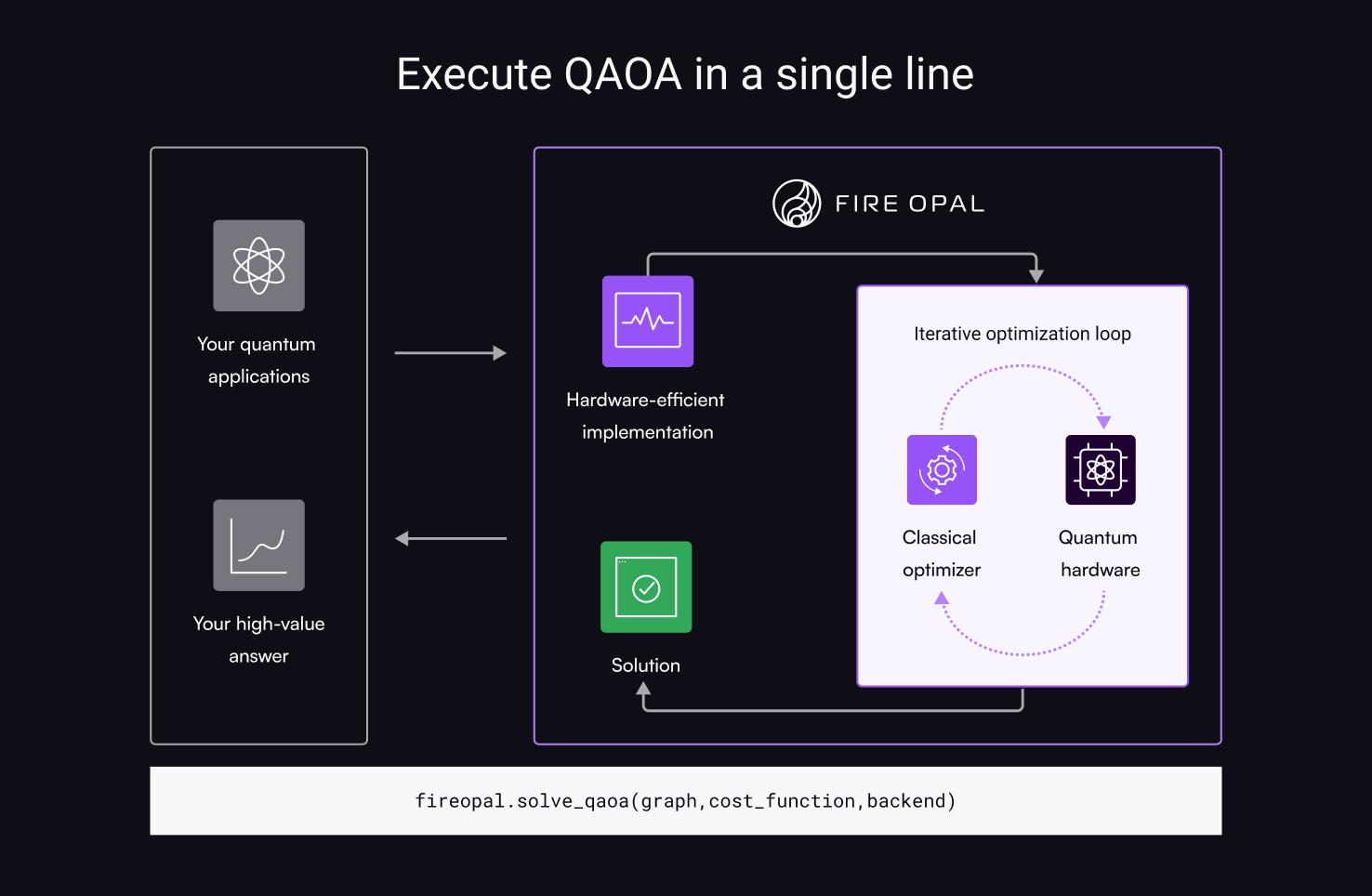
Once we constructed the problem in the right way, this algorithm was executed using the Q-CTRL automated compilation and error suppression pipeline, allowing us to conduct preliminary quantum hardware tests and achieve high-quality results on an IBM Quantum 127-qubit device.
Leveraging error suppression to overcome hardware limitations
The biggest impediment to realizing quantum advantage for this or any other problem is the need to deal with errors in quantum computing hardware. Executing an algorithm directly on bare metal hardware produces results subject to frequent errors and failures - for industrial-scale problems this results in performance that is generally only marginally better than randomly guessing solutions.
To counter this, we can combine our new approach to quantum optimization with Q-CTRL’s proprietary automated error suppression pipeline! Even in the preliminary trials, we ran in Phase 1 of our program, we were able to show massive enhancements to solution quality by combining Q-CTRL error-suppression technology with IBM hardware.
We’re now scaling our solution to larger problems in the next phase - tackling error suppression at each stage of the solution stack will be essential and at the core of our approach.

Looking ahead to Phase II and beyond
Quantum computing is at an exciting stage where hardware devices are approaching the size and performance needed to solve problems that industry values most. Early logistics applications will occur where small improvements over existing solutions enable significant environmental and economic benefits across an entire supply chain. Over the coming decade, we foresee enterprises spanning aviation, automotive, and beyond applying early quantum computing as part of production solutions for key optimization problems. The Airbus and BMW quantum mobility challenge is a great example of how end-users are now driving high-impact outcomes.
As a finalist in the challenge we are now collaborating with technical experts from Airbus and BMW to ensure that our solver is able to deliver maximum value. This involves exploring tradeoffs in optimizing for different goals like reduced emissions, minimal transportation costs, and fast lead times, as well as introducing extra practical considerations into the problem model such as ensuring a fair manufacturing distribution amongst different regions and suppliers. By introducing these extra considerations, we ensure our methodology will accurately model the real-world problems faced in industrial supply chain optimization.
After integrating these new problem attributes into our approach, we will demonstrate our solver’s performance on current quantum computers through detailed benchmarking at large scales, and finally present the future prospects and roadmap to practical solution enhancement by investigating the scalability of our algorithm in delivering large-scale optimization solutions using quantum computing.
The countdown is on to Q2B Silicon Valley where the winners of the Quantum Mobility Quest will be announced. Visit our booth to learn more about our error suppression technology and how it can help you solve utility-scale problems.
Discover more examples of our work in developing quantum computing solutions for logistics optimization with the Australian Army and Transport for NSW.