Making quantum useful with AI-powered infrastructure software


The annual APS March Meeting is just around the corner. We’re excited to bring our cutting-edge research to the world’s largest physics community event again this year, and to showcase our exceptional products for R&D professionals like you.
Our team of quantum control experts will present six insightful talks across a range of new methods and techniques that have enabled us to achieve the record-breaking results we’ve demonstrated in both quantum computing and quantum sensing.
Mark your calendars for the sessions below where we’ll present the AI-driven techniques that power our software to push quantum computers to the absolute limits.
Characterizing quantum hardware
- M50.00006 Fast full-device characterization and gate calibration with a graph-based scheduler
Improving quantum algorithm performance with error suppression
- N50.00012 Improving algorithmic performance using hardware efficient gates
- F51.00008 A graph-based embedding of dynamical decoupling for quantum computing
- D52.00001 Resource efficient transfer learning approach for error mitigation of quantum circuits at scale
Designing efficient hybrid algorithms
- K51.00009 Enabling hybrid algorithms on utility-era devices via error suppression
- Q51.00002 Quantum algorithm co-design for field-testing the path to advantage
👀 Psst… we will be unveiling several exciting front-end integrations with key partners in the coming weeks - from control electronics to quantum algorithm IDEs! Stay tuned for more details.
Transforming the utility and performance of quantum computers with infrastructure software
Visit our booth #705 to discover how our infrastructure software for hardware design and automation and performance management, along with our world-class quantum education products can supercharge your quantum research and teaching programs, and enable you to gain a strategic first-mover advantage.
Whether you are a researcher, algorithm designer, educator, or a newcomer to the field, we make quantum technology useful for you - all through software.
Build the quantum platforms of the future
Boulder Opal is the world’s first design-and-test EDA for the quantum era! Comprised of flexible and powerful tools for hardware design, automation, and performance optimization, Boulder Opal enables quantum R&D teams to accelerate the advancement of quantum computing and quantum sensing at scale.
By combining cutting-edge research capability and flexibility with cloud-accelerated performance, we’re helping research teams accomplish more in less time.
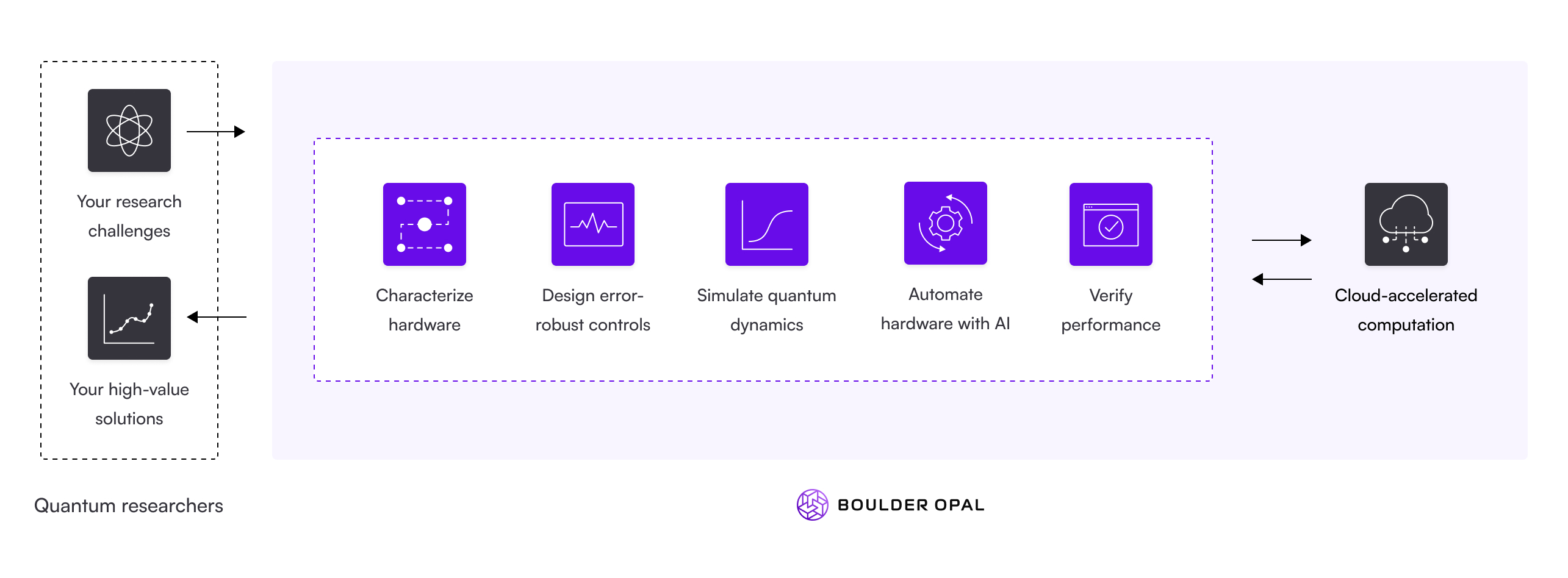
👉 Try Boulder Opal for free and discover how it can help unlock the full potential of your quantum R&D.
Find true value from quantum algorithms
Fire Opal delivers effective error suppression technology for quantum computers as a simple, fully automated solution suitable for any user. Quantum algorithm developers and researchers can use Fire Opal to deploy fully autonomous error suppression in cloud quantum computers - and boost result quality by thousands of times!
Get meaningful results from real hardware with automated error suppression, which enables you to run deeper and wider circuits while maintaining accuracy. Leverage out-of-the-box application solvers to achieve valuable outcomes in your most important use cases, such as logistics optimization and job scheduling, without worrying about hardware noise - or even programming quantum circuits!
👉 Try Fire Opal for free and run your most exciting algorithms with up to 127 qubit devices today. Simply sign up, install, and run your circuit to experience better results.
This technology can also be used to unleash latent hardware potential in your quantum computing platforms. Chat with us about integrating our error suppression software natively into your quantum systems - just like we recently did with IBM Quantum services.
Prepare your students or team for a quantum career
Educators, higher education institutions, and skills training providers can supercharge quantum computing curriculum and delivery with Black Opal, the best interactive platform for quantum education.
Get everything you need to build an introductory quantum course in one place or augment your existing quantum education initiatives with interactive self-paced learning.
👉Contact us to book a demo or try Black Opal for free.
Schedule a meeting with our executives at APS
No matter your background, we can make quantum technology useful for you. Book a meeting with senior leaders from our research and product teams attending the APS March Meeting to discuss your needs.
If you’re not attending the conference in person, contact us to schedule a meeting or sign up for our newsletter to stay up-to-date on research and product developments.
APS scientific abstracts
Monday, March 4
D52.00001 Resource efficient transfer learning approach for error mitigation of quantum circuits at scale
Yuval Baum, Gavin S Hartnett, Nuiok Dicair
Large-scale fault-tolerant quantum computers are likely to enable new solutions for problems known to be hard for classical computers. This potential is tempered by the reality that hardware is exceptionally fragile and error-prone, forming a bottleneck in the development of novel applications. While error suppression techniques can dramatically boost algorithmic performance, inherent and irreversible errors, such as T1 processes, limit the ability of achieving quantum utility at scale.
Error mitigation techniques provide a route to go beyond that limit at the price of additional resources overhead. Some of these approaches, such as probabilistic error cancellation (PEC), aim to collect a full description of the noise model to facilitate noise suppression. However, this requires over-sampling, which is costly to acquire and scales poorly as the number of qubits increases. In this work, we develop a new, scalable error mitigation approach based on training machine learning models on real device data. We focus on problems where the quantity of interest is a set of expectation values, such as quantum simulations or variational quantum eigensolvers (VQE).
Using non-parametric ensemble models, we machine-learn the approximate inverse noise map for a subset of quantum observables. This is achieved by training models on a closely related family of circuits which are efficiently simulable, and then using transfer learning to apply the learned inverse noise map to the circuits of interest. As a demonstration of our approach, we estimate Pauli observables and show a consistent ability to mitigate errors with a significantly reduced runtime compared to established mitigation techniques such as PEC and zero-noise extrapolation (ZNE).
Tuesday, March 5
F51.00008 A graph-based embedding of dynamical decoupling for quantum computing
Paul Coote, Roman Dimov, Gavin Hartnett, Yuval Baum
Dynamical decoupling (DD) is a key error suppression technique for quantum computing. Ideally, DD suppresses both single-qubit phase errors and unwanted crosstalk between coupled qubits during idle delays. The precise timing of the DD gates or pulses affects the efficiency of error suppression and ultimately the circuit fidelity. Here, we present an automated and efficient method for determining an embedding of DD to suppress phase and cross-talk errors for an arbitrary input circuit.
Our method relies on representing the DD embedding task as a graph, and using the structure and properties of the graph to determine an order in which each idle delay should be addressed. This ordering allows optimal DD embeddings to be found analytically (without using numerical optimization). Our procedure finds a tailored DD scheme that refocuses all quasi-static ZI and ZZ error terms, up to minor unavoidable rounding errors due to device timing constraints.
Moreover, the protocol uses a small number of gates compared to alternative techniques. Our method provides a clear boost to overall empirical algorithmic success probability compared to other DD embedding schemes. We present the DD embedding algorithm as well as side-by-side empirical tests with other known DD embedding schemes.
Tuesday, March 5
K51.00009 Enabling hybrid algorithms on utility-era devices via error suppression
Pranav S Mundada, Yulun Wang, Yuval Baum, Natasha Sachdeva, Hank Greenburg
While scalable and fault-tolerant quantum computers are currently out of reach, hybrid quantum-classical algorithms provide a path towards achieving quantum advantage for certain types of optimization problems on utility-era devices. Yet, errors and imperfections in existing quantum computers degrade the performance of these algorithms, rendering them unavailing.
Various statistical techniques, such as ZNE and PEC, have been used to address this challenge. However, these methods can be applied to only a small subset of problems, and introduce an extensive sampling overhead, increasing the time and cost required to complete these tasks. We show that a deterministic error suppression workflow, with no overhead, improves the performance of hybrid algorithms on currently available quantum hardware by addressing both the quality of implementation of individual circuits and the effectiveness of the classical optimization loop.
In this talk, we review the different parts of our workflow and demonstrate its effectiveness via the implementation of QAOA on real devices. We show orders of magnitude improvement in performance alongside a substantial reduction in the resources needed for achieving these tasks. In particular, we show that we can consistently, with over 99% success rate, correctly solve combinatorial optimization problems, such as MaxCut and Min Vertex Cover, using minimal resources on >40Q superconducting quantum devices.
Wednesday, March 6
M50.00006 Fast Full-Device Characterization and Gate Calibration with a Graph-Based Scheduler
Ayush M Pancholy, Aaron Barbosa, Varun Menon, Shobhan Kulshreshtha, Yuval Baum, Pranav S Mundada, Marti Vives
Reliable calibration of all quantum operations is crucial in obtaining the best device performance limited only by the qubit coherences. Traditionally, calibration is done using slow, resource-intensive model-based experiments executed in a manual or semi-automated operation requiring expert supervision. Due to the inadequacy of the device models for fast gates, the above procedure is often unable to achieve the best device performance.
This talk presents a system-wide tune-up approach for quantum devices. We enable fully autonomous bring-up from a cold start by leveraging AI tools that directly interact with the hardware and control technology. We developed a graph-based scheduler that seamlessly combines parallelized device characterization and gate calibration. Our protocol bootstraps the noisy device parameters obtained from coarse model-based device characterization with model-free agents to realize optimal gates. This enables us to reduce the number of measurements by over 10x compared to the standard scripted multi-dimensional scans. We experimentally demonstrate complete device tune-up, quickly obtaining high-fidelity gate and measurement waveforms compared to slower traditional calibration methods. Subsequent benchmarking verifies that the device operation fidelity is coherence-limited.
Wednesday, March 6
N50.00012 Improving algorithmic performance using hardware efficient gates
Yulun Wang, Ashish Kakkar, Samuel Marsh, Hank Greenburg, Yuval Baum, Pranav S Mundada
Useful quantum algorithms that utilize only the standard entangling gates like CNOT, iSWAP, etc. fail to produce meaningful results on the NISQ devices. Superconducting circuits provide a diverse variety of native multi-qubit interactions depending on the device architecture and gate-drive scheme. The calibration of high fidelity parameterized gates and the construction of an efficient circuit compilation scheme that leverages the richer gate set are open problems.
In this talk, we present an efficient method for optimizing a selected set of system-wide entangling gates. These gates can be dynamically used online to create short-duration, high-fidelity parametric gates with arbitrary angles, all while demanding minimal calibration resources. To ensure the optimal incorporation of these gates in algorithms, we built a specialized compilation procedure that automatically finds and replaces optimizable patterns in quantum circuits. We demonstrate that our pulse-efficient gate construction and calibration technique enables both a higher fidelity and shorter duration than the standard implementation.
This leads to enhanced performance across several key quantum algorithms, including QFT, Trotterized time evolution, and QAOA. For instance, when running a 7-qubit MaxCut QAOA, the default circuit execution on a cloud-accessible quantum computer fails to give the correct bit string as the mode of the output distribution while our approach achieves the correct answer with 99% probability. These results signify the importance of both the hardware efficient gates and deterministic error suppression for making quantum devices useful.
Wednesday, March 6
Q51.00002 Quantum algorithm co-design for field-testing the path to advantage
Gavin S Hartnett, Andre Carvalho, Samuel Marsh, Christopher Bentley
To help end users to appreciate both the current status and the potential future impact of quantum computing, providing practical-scale solutions that leverage quantum resources is a powerful demonstration. These demonstrations ideally satisfy three criteria: (1) a current quantum computation at a non-trivial scale, (2) a smooth scaling approach such that the quantum computation can measurably scale towards a target threshold for potential quantum advantage, and (3) algorithm design that minimizes the most challenging resources for a given hardware.
We illustrate this approach using a scheduling and routing scenario for the Australian Army: our hybrid quantum algorithm provides solutions for full-scale use-cases and features (1) a current quantum routing sub-routine for ~6 vehicles on several available routes, (2) a problem decomposition method that allows scaling of the number of vehicles towards the classically-challenging threshold at ~100 vehicles, and (3) resource requirements of only sparse two-qubit connectivity outside local qubit clusters and circuit duration less than 1ms for classically-challenging problems (within challenging but achievable T1 limits) on superconducting devices.
We highlight the impact of Q-CTRL’s error-reducing infrastructure software, which increases current high-quality solution size (2X larger than direct hardware deployment), provides >6X improvement in time-to-solution, and reduces the device T1 limit required for large-scale problems by ~20%.