Bringing our cutting-edge quantum technology research to APS March Meeting 2023

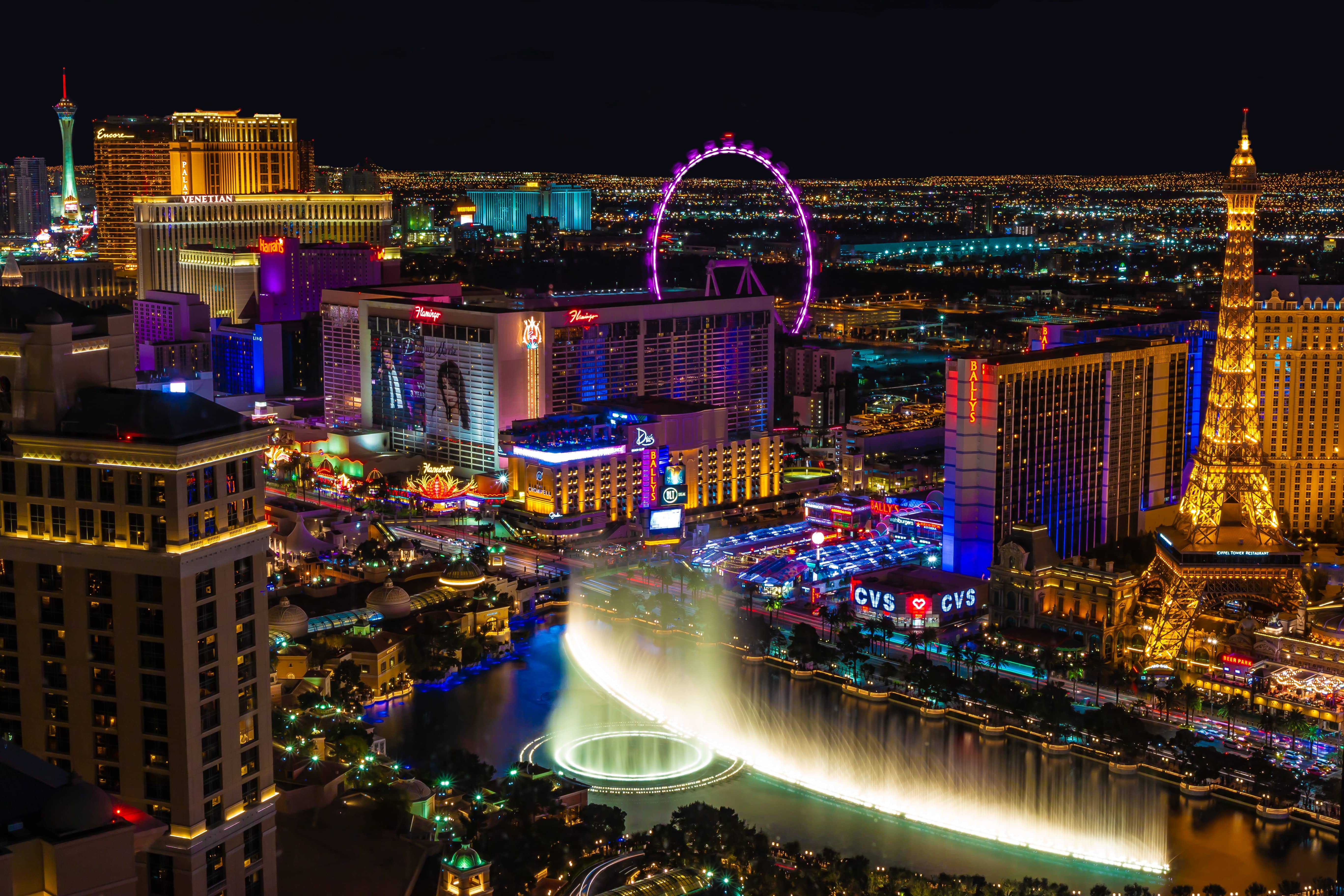
It’s that time of year again! Time flies when you’re having fun driving quantum technology research and product innovation.
Following a year of extraordinary technical developments, we’re thrilled to be bringing our cutting-edge research to the world’s largest physics community event again this year at APS March Meeting 2023.
Our team of quantum control experts will present eight exciting talks across a range of topics in quantum computing and quantum sensing. We’ll blow your mind with some extraordinary results applying AI to quantum hardware and pushing quantum computers to the absolute limits. You won’t want to miss this lineup 👇
Improving quantum algorithm performance with error suppression
- G73.002 Improving algorithmic performance of tunable superconducting qubits using deterministic error suppression
- G70.007 Using machine learning for autonomous selection of optimal qubit layouts on quantum devices
- K70.011 Efficient autonomous system-wide gate calibration
Making Quantum Error Correction practical
- S72.015 Improving syndrome detection using quantum optimal control
Designing efficient hybrid algorithms
- D58.008 Maximizing success and minimizing resources: An optimal design of hybrid algorithms for NISQ era devices
- G73.008 Eliminating overhead: Improving the performance of hybrid algorithms using deterministic error suppression
Characterizing quantum hardware
- B72.009 Optimized Bayesian system identification in quantum devices
Ruggedizing quantum sensors
- N65.001 Improving the performance of cold-atom inertial sensors and gravimeters using robust control
Download the Q-CTRL APS presentation abstracts schedule.
Meet our team at APS
Be sure to stop by booth #506 to discover how we can help you move your quantum R&D faster with the most advanced characterization, optimization, and automation tools for quantum research.
Psst … we’ll also be unveiling some exciting new capabilities to help you scale from one to 100 qubits!
If you’re not attending in-person this year, you can contact us directly to discuss your needs or sign-up to our newsletter to stay up-to-date on our latest research and product developments.
Build the quantum platforms of the future
Boulder Opal is a versatile Python package that provides everything a research and development team needs to accelerate advancement of quantum computing and quantum sensing at scale.
It delivers comprehensive functionality enabling you to characterize hardware, design error-robust controls, simulate complex quantum dynamics, automate hardware with AI, and verify performance.
And Boulder Opal is quick and powerful! With automatic cloud-compute acceleration you can reduce wall-clock runtimes for complex computations by almost 100x and handle system dimensions that break other packages.
You can save hours by eliminating manual tasks and push your research further than you thought possible.
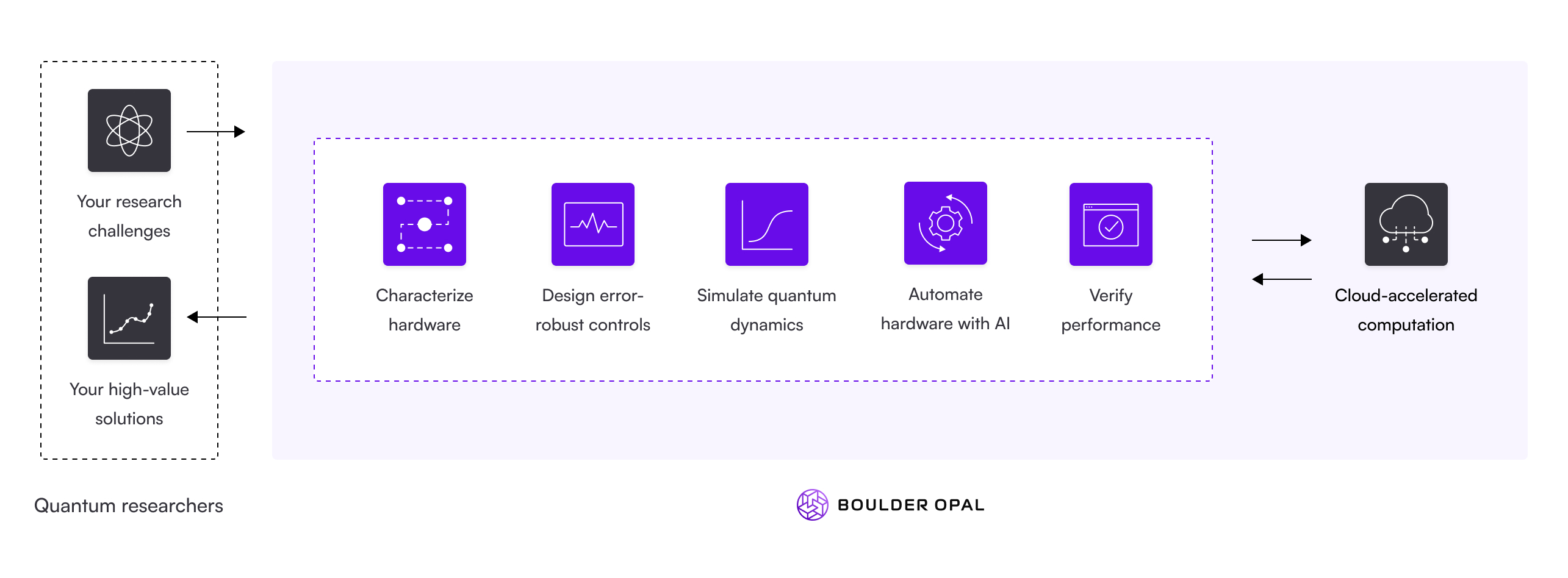
Discover how Boulder Opal can help unlock the full potential of your quantum R&D.
APS presentation abstracts
Monday, March 6
Optimized Bayesian system identification in quantum devices
B72.009 Dr. Ashish Kakkar, Senior Scientist, Quantum Error Correction
Identifying and calibrating quantitative dynamical models for physical quantum systems is critical in the development of a quantum computer. We present a closed-loop Bayesian learning algorithm for estimating multiple unknown parameters in a dynamical model, using optimized experimental “probe” controls and measurement. The estimation algorithm is based on a Bayesian particle filter, and is designed to autonomously choose informationally-optimized probe experiments with which to compare to model predictions. In both simulated and experimental demonstrations, we see that successively longer pulses are selected as the posterior uncertainty iteratively decreases, leading to an exponential scaling in the accuracy of model parameters with the number of experimental queries. In an experimental calibration of a single qubit ion trap, we achieve parameter estimates in agreement with standard calibration approaches but requiring ∼ 20x fewer experimental measurements. We also demonstrate the performance of the algorithm on multi qubit superconducting devices, demonstrating the flexibility of these techniques.
Maximizing success and minimizing resources: An optimal design of hybrid algorithms for NISQ era devices
D58.008 Dr. Yulun Wang, Senior Scientist, Quantum Control
Hybrid algorithms can potentially deliver quantum advantage in problems from physics, chemistry and optimization. Practical implementations on current available quantum hardware are still challenged by optimization inefficiency, poor scalability, runtime overhead and inaccuracy. In this work, we redesign the QAOA and VQE algorithm systematically to efficiently improve the success rate and accuracy while using minimal resources. By automating and optimizing the ansatz selection, cost function and parameters optimization we can reduce the complexity of the classical component of hybrid algorithms, leading to a much faster, stable and consistent convergence. We show the redesigned QAOA procedure exhibiting 6x improvement in success probability solving Max-Cut problem with at least 3 times less function calls compared to commonly used randomized initialization methods. We also demonstrate an improved accuracy and convergence speed from the redesigned VQE procedure. Finally, we demonstrate further algorithmic improvements achieved by applying our deterministic error-suppression workflow on NISQ hardware, which provides the hybrid algorithm a robust noise resistance and enables the scalability to larger devices.
Tuesday, March 7
Eliminating overhead: Improving the performance of hybrid algorithms using deterministic error suppression
G73.008 Dr. Smarak Maity, Senior Software Engineer, Quantum Control
Large-scale fault-tolerant quantum computers will enable new solutions for problems known to be hard for classical computers. While scalable and fault-tolerant quantum computers are currently out of reach, hybrid quantum-classical algorithms provide a path towards achieving quantum advantage for certain types of optimization problems on NISQ devices. Errors and imperfections in existing quantum computers degrade the performance of these algorithms. Various statistical techniques have been used to address this issue, including zero noise extrapolation and random compilation methods such as Pauli twirling. These techniques introduce additional sampling overhead, increasing the hardware execution time required to complete the tasks. In this talk, we show that a deterministic error suppression workflow improves the performance of hybrid algorithms on currently available quantum hardware. This is done by improving the performance of arbitrary quantum circuits on the hardware, without introducing any additional overhead. We demonstrate the effectiveness of our tools via the implementation of QAOA and VQE on real devices. Our workflow improves the structural similarity index (SSIM) of the energy landscape (compared to the ideal landscape) by 28x for a 5-qubit QAOA problem, and improves the mean energy deviation (from the ideal energy) by 5x for a 6-qubit VQE problem. In this way, our methods render non-working algorithms into useful ones, while keeping the required hardware time low.
Using machine learning for autonomous selection of optimal qubit layouts on quantum devices
G70.007 Aaron Barbosa, Senior Scientist, Quantum Control
In recent years, the experimental performance of quantum algorithms on real hardware has increased substantially. This is due not just to advancements in quantum hardware, but also the development of a vast array of classical preprocessing techniques. Advancements in compilation, layout selection, and quantum control have been used to push the performance of quantum devices right up to the hardware limit. Layout selection is the process by which virtual qubits in a quantum circuit representation are mapped to a subset of physical qubits on a quantum computer. Choosing a suboptimal qubit layout can be very detrimental for the performance of a quantum algorithm. On NISQ-era quantum devices, each qubit often has a unique coherence time, readout error, gate fidelity, and so on. Before executing a circuit, experts need to carefully balance these traits to select an optimal qubit layout, thus hopefully maximizing the performance of the device. In this work, we explore the effects of layout selection on quantum algorithm performance. We propose a set of heuristics for performing layout selection on quantum devices. We observe that an optimal layout can increase algorithm success probability up to 3x when compared to a suboptimal layout. Using our methods, we are able to achieve substantial improvements on real hardware across a wide range of quantum algorithms such as BV, QAOA, and QFT. We also demonstrate that our tools vastly outperform existing solutions.
Improving algorithmic performance of tunable superconducting qubits using deterministic error mitigation
G73.002 Varun Santhosh Menon, Research Scientist, Quantum Control
The largest NISQ era devices currently available utilize superconducting architectures. Flux tunable superconducting qubits in particular help avoid frequency collisions in large devices, and can utilize fast gates and measurement schemes. However, such architectures are affected by a higher sensitivity to flux noise, affecting both single and two qubit gate fidelity, in addition to decoherence and readout errors that inhibit the performance of all NISQ era devices, ultimately leading to suboptimal accuracy on even few qubit algorithms. In this work, we show how our deterministic error mitigation pipeline improves the performance of superconducting hardware on algorithmic benchmarks. In particular, we utilize intelligent quantum compilation and physics-aware circuit layout selection strategies, optimized single and two qubit gate calibration, measurement readout error mitigation, and correlated dynamical decoupling, to reduce errors at every stage of the quantum hardware stack. We demonstrate these methods on the Rigetti quantum computer, and show over a 1000x improvement in accuracy on up to 15 qubit Bernstein-Vazirani and 30 X on up to 7 qubit Quantum Fourier Transform circuits. We also show that our calibration methods efficiently improve both single and two qubit gate fidelities across the device, leading to improvements of more than 7 X over the default fidelities. Furthermore, we show that our methods suppress idling errors and crosstalk across the device, leading to a significant increase in quantum volume of the quantum computer.
Efficient autonomous system-wide gate calibration
K70.011 Marti Vives, Research Scientist, Quantum Control
An important step in the path towards full-fledged quantum computation is the ability to maintain high fidelity gates across a full device and across time. Current NISQ devices are error-prone and fragile, suffering from a wide range of errors, including dephasing, leakage and cross-talk, which may lead to low quality gates and drift in gate performance over time. In this talk we present a quantum control pipeline, based on Clifford circuits, to efficiently perform a system-wide calibration through parallel and automated-closed loop optimization of microwave pulses. Widely used methods such as randomized benchmarking tend to underestimate coherent errors, while methods that are based on process tomography require significant resources. Our method maintains high sensitivity to both coherent and non coherent errors while being machine efficient, allowing full device calibration in a matter of minutes. We show that our implemented single and two qubit gates yield fidelities near the T1 limit, and demonstrate our methods across different hardware providers and across devices, showing over 7x improvement in fidelity compared to default gates.
Wednesday, March 8
Improving the performance of cold-atom inertial sensors and gravimeters using robust control
N65.001 Dr. Russell Anderson, Head of Quantum Sensing
Large momentum transfer (LMT) atom-optical beam splitters and mirrors—implemented via Bragg diffraction—increase the space-time area enclosed by atom interferometers, offering a promising means of improving the precision of cold-atom inertial sensors. However, to date LMT has not improved absolute cold-atom inertial sensitivity, due to the stringent velocity-selectivity of LMT Bragg pulses making high contrast atom interferometry with appreciable atomic flux extremely challenging. In this work, I will present theoretical and experimental results showing how error-robust quantum control can be used to overcome these traditional limitations on LMT Bragg atom interferometry. Our unique approach to quantum hardware optimization and operation allows us to design LMT pulses with increased velocity acceptance and improved robustness to laser intensity inhomogeneity. We demonstrate that our control solutions provide > 10X improvement in measurement sensitivity over Gaussian Bragg pulses at 102 ћk momentum separations, and further enable operation at longer interrogation times and without velocity selection of the initial cold thermal atomic source. Our results show how quantum control at the software level can improve future cold-atom inertial sensors, enabling new capabilities in navigation, hydrology, and space-based gravimetry.
Thursday, March 9
Improving syndrome detection using quantum optimal control
S72.015 Dr. Gavin Hartnett, Senior Scientist, Quantum Control
In order to achieve fault-tolerant quantum computation, it will be necessary to continuously correct errors using quantum error correcting codes. To meet this goal, it will be crucial to continue to develop better codes and decoders, as well as to improve the implementations of basic building blocks of error correction, such as stabilizer circuits and parity check operations, on real NISQ-stage devices available today. Here, we present recent results along these lines. First, we demonstrate that the fidelity of small-scale quantum error correcting codes can be improved using our deterministic error-suppression workflow, which is designed to reduce non-Markovian noise from a variety of sources using error-aware compilation, system-wide gate optimization, dynamical decoupling, and measurement-error mitigation. Results obtained using our workflow are consistently better than the default pipeline: we find improved error detection success rates which are 2.5 and 3.3 times higher compared to the default approach. Second, we introduce a faster implementation of the 2-qubit parity check operation and then use quantum optimal control techniques to calibrate this operation. This approach allows for improved parity checks that have both a higher fidelity and shorter duration than the standard implementation based on multiple two-qubit controlled gates.