Achieve ~100x efficiency improvements in quantum R&D with automated hardware characterization

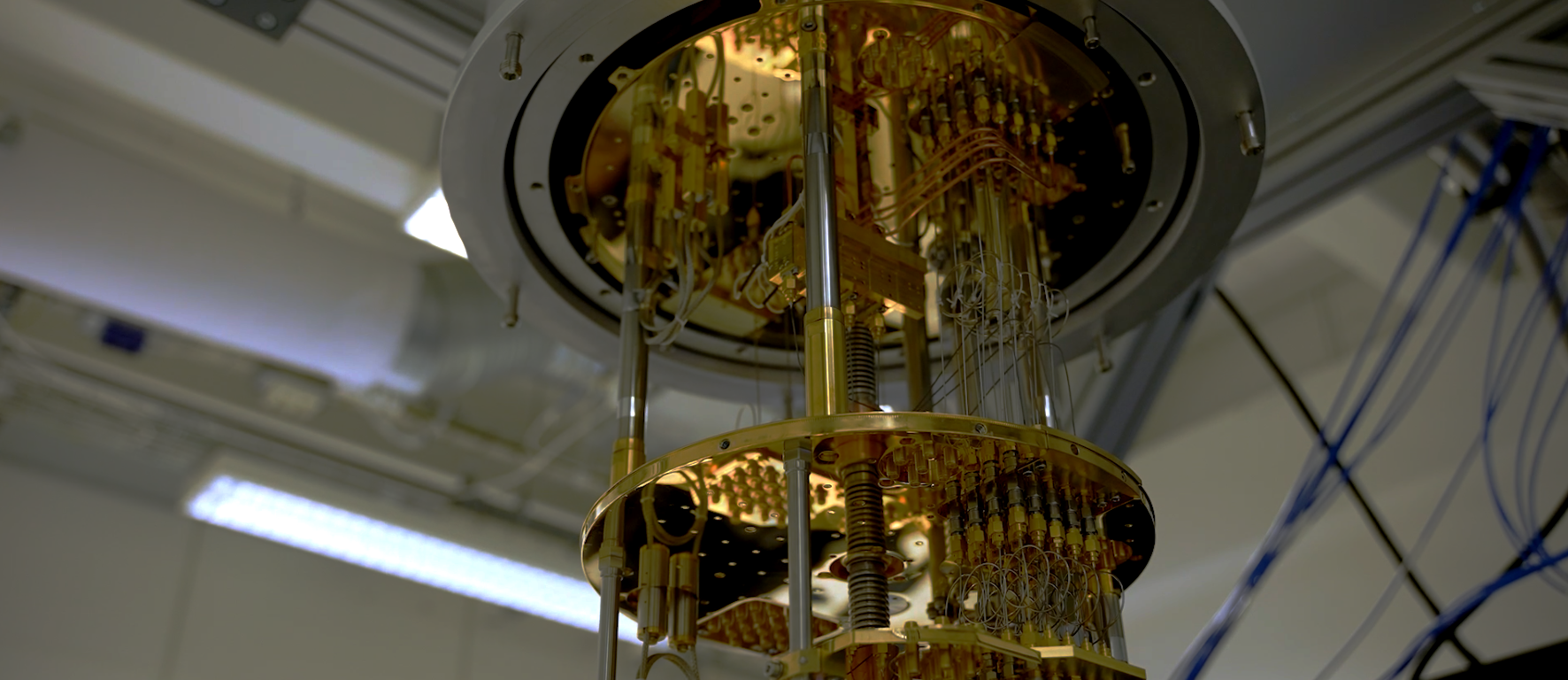
Hardware characterization is a known pain point for any quantum R&D team. Researchers need to learn hardware parameters and identify imperfections and deviations from design specifications in order to operate their systems at peak performance. In our recent survey of experimentalists, we found that 50% of respondents ranked finding new and more efficient ways to characterize their devices as their top priority.
Fortunately, our infrastructure software solution can solve your most pressing characterization challenges! In our latest webinar, we show you how to characterize your quantum devices for quantum computing and sensing faster and easier with automated system identification.
We focus on our new research results in collaboration with the Quantum Control Laboratory at the University of Sydney where we characterized a trapped ion experiment 93x more efficiently than standard techniques, using 60x fewer measurements and even delivering a 55% improvement in precision of the estimated parameters. The whole routine is completely autonomous meaning you can focus on the physics questions where your attention is needed.
If you want to design new robust controls for high fidelity operations, or identify difficult-to-measure Hamiltonian parameters to improve system models, this automated approach to characterization is nearly optimal. A manuscript is available on the arXiv which covers in detail all the results we have presented.

Under the hood of optimal characterization
So, how does this optimal characterization work? At the heart, it is powered by a new algorithm called OBSID, which stands for Optimal Bayesian System Identification.
OBSID is totally autonomous - the algorithm determines what measurements to perform, how to process them, and how to iteratively improve parameter estimates. Using OBSID you create a probabilistic model for the possible values of the parameters Hamiltonian, and then update this model after every measurement using Bayes rule. This part of the algorithm is pretty standard.
The real magic is how OBSID automatically picks the probe pulses which will give you the most information about your system - even in a large Hilbert space with many available controls.
If that seems complicated, don’t worry! You don’t have to know the details of how the algorithm works, our expert team can help you get it up and running on your device in three easy steps:
Step 1: Define your best guess for the Hamiltonian of your system (other representations, like master equations, can also work). You can add terms for potential noise sources and include more complex terms in the Hamiltonian which you don’t know how to characterize. The OBSID algorithm will automatically determine the optimal strategy to characterize all parameters in the Hamiltonian: all you have to do is input the model.
Step 2: Run the experiment iteratively. The OBSID algorithm will automatically create probe pulses using the available control knobs in your system that extract the maximum information from the experiment. After each run the measurement results must be fed back to the algorithm (handled automatically with our tools). OBSID uses this information to update its estimate of the Hamiltonian, and determine the next probe pulse to use. This loop is repeated until it is able to find the true parameters up to precision specified by the user.
Step 3: Access the results. Once the experiment is complete, the algorithm will return the true Hamiltonian including its best estimates for all the parameters in the Hamiltonian you specified and the associated precision.
This iterative characterization process is completed automatically (you don't have to change anything) and is optimal, meaning the number of measurements required is the minimum possible.
In our tests with the Quantum Control Lab, the number of measurements required was reduced by about 60x relative to the best known "brute force" characterization techniques. Hours of manual tuneup could be reduced to just a few minutes, with no human intervention. Now imagine achieving those savings over dozens of hardware parameters to understand how much time you could save in your experiments!
Cutting edge research driving product innovation
The new OBSID algorithm is coming soon to Boulder Opal - a Python package that provides everything a research team needs to automate and optimize the performance of hardware for quantum computing and quantum sensing at scale. The toolset is connected to our powerful cloud-compute infrastructure to boost its numerical performance, getting you the right solutions faster.
These new features are a powerful complement to the ability to perform detailed noise spectroscopy in hardware that we discussed in a previous webinar. There we used our noise spectroscopy tools to accurately characterize frequency noise during quantum logic gates via displaced cat states in a trapped ion experiment - we even identified lab sources of noise that could be eliminated by simple hardware changes. For more details, see our publication in Physical Review Letters.
And if you're looking to take hardware characterization to a new level of abstraction, we also have a fully automated suite of tools for characterizing errors at the algorithmic level; this work is best summarized in our recent manuscript where we demonstrated that appropriate hardware characterization and targeted error suppression could improve the performance of quantum algorithms executed on commercial quantum computers by over 1,000x.
Get started today
We hope that this webinar has given you insight into how the new OBSID characterization process works, and ideas for how it can be used on your quantum device to help you move faster.
Get in touch with our expert team to learn more, gain early access to this capability, and explore how we can help accelerate your research.